Our Research
Introduction
Pacmed is involved in applied research in areas that are relevant to the use of AI and ML in healthcare, paying special attention to the preconditions for careful and responsible use of state-of-the-art technology. In addition, we strive for scientific validation of all steps of development and implementation, as well as education on all aspects of data-driven care. Therefore, Pacmed explicitly seeks collaboration with partners to ensure that the results of our research benefit everyone.
Pacmed Critical
Our research around Pacmed Critical covers the development of the machine learning model at Amsterdam UMC location VUmc, the external validation at LUMC and ETZ hospitals and the development and testing of the decision support software for ICU discharge.
When, where, who, what, and why? The five Ws of workflow analysis for implementing an AI decision support tool at the intensive care
Anne A.H. de Hond, Suzanne Vosslamber, Sanne Lange, Friso Engel, Mette Lindhout, Puck Noorlag, Ewout W. Steyerberg, Giovanni Cinà, M. Sesmu Arbous.
Human Factors in Healtcare. 2025 Feb;19 (7)
Explainable Machine Learning on AmsterdamUMCdb for ICU Discharge Decision Support: Uniting Intensivists and Data Scientists
Thoral PJ, Fornasa M, de Bruin DP, Tonutti M, Hovenkamp H, Driessen RH, Girbes AR, Hoogendoorn M, Elbers PW.
Critical Care Explorations. 2021 Sep;3(9)
Predicting readmission or death after discharge from the ICU: external validation and retraining of a machine learning model
de Hond, A. A., Kant, I. M., Fornasa, M., Cinà, G., Elbers, P. W., Thoral, P. J., ... & Steyerberg, E. W.
Critical care medicine (2023), 51(2), 291-300.
Transferring clinical prediction models across hospitals and electronic health record systems. [link]
Curth A, Thoral P, van den Wildenberg W, Bijlstra P, de Bruin D, Elbers P, Fornasa M.
Joint European Conference on Machine Learning and Knowledge Discovery in Databases 2019 Sep 16 (pp. 605-621).
The Potential Cost-Effectiveness of a Machine Learning Tool That Can Prevent Untimely Intensive Care Unit Discharge.
de Vos J, Visser LA, de Beer AA, Fornasa M, Thoral PJ, Elbers PW, Cinà G.
Value in Health. 2022 Oct 22.
Intensive Care Unit Physicians’ Perspectives on AI–Based Clinical Decision Support Tools: Pre Implementation Survey Study
van der Meijden SL, de Hond AAH, Thoral PJ, Steyerberg EW, Kant IMJ, Cinà G, Arbous MS
JMIR Hum Factors 2023;10:e39114
Development and clinical implementation of real-time decision support tools for ICU discharge
P.J. Thoral, Anne de Hond, C.M. Sauer, D.P. de Bruin, M. Fornasa, Sesmu Arbous, Giovanni Cinà, P.W.G. Elbers,
Journal of Critical Care, Volume 81, 2024,
COVID Predict and ICU data
Our research around Covid Predict and ICU data covers the development of the Dutch national COVID-19 icu patients database and its successor: ICU data, and the research around prognosis and treatment of patients admitted with COVID-19 in the Netherlands.
The Dutch Data Warehouse, a multicenter and full-admission electronic health records database for critically ill COVID-19 patients
Fleuren LM, Dam TA, Tonutti M, de Bruin DP, Lalisang RC, Gommers D, Cremer OL, Bosman RJ, Rigter S, Wils EJ, Frenzel T.
Critical Care. 2021 Dec;25(1):1-2.
Predictors for extubation failure in COVID-19 patients using a machine learning approach
Fleuren LM, Dam TA, Tonutti M, de Bruin DP, Lalisang RC, Gommers D, Cremer OL, Bosman RJ, Rigter S, Wils EJ, Frenzel T.
Critical Care. 2021 Dec;25(1):1-0.
Risk factors for adverse outcomes during mechanical ventilation of 1152 COVID-19 patients
Fleuren LM, Tonutti M, de Bruin DP, Lalisang RC, Dam TA, Gommers D, Cremer OL, Bosman RJ, Vonk SJ, Fornasa M, Machado T.
Intensive care medicine experimental. 2021 Dec;9(1):1-5.
Large-scale ICU data sharing for global collaboration: the first 1633 critically ill COVID-19 patients in the Dutch Data Warehouse
Fleuren LM, de Bruin DP, Tonutti M, Lalisang RC, Elbers PW.
Intensive care medicine 2021 Apr;47(4):478-81.
Variation in Ventilator Settings for Coronavirus Disease 2019 Acute Respiratory Distress Syndrome
Dam TA, de Grooth HJ, Klausch T, Fleuren LM, de Bruin DP, Entjes R, Rettig TC, Dongelmans DA, Boelens AD, Rigter S, Hendriks SH.
Critical care explorations. 2021 Oct;3(10).
The effect of prone positioning on mechanically ventilated COVID-19 patients
Izdebski A, Thoral PJ, Lalisang RC, McHugh DM, Entjes R, van der Meer NJ, Dongelmans DA, Boelens AD, Rigter S, Hendriks SH, de Jong R.
arXiv preprint arXiv:2109.06707. 2021 Sep 14.
Responsible AI in Healthcare
Our research around responsible AI in healthcare covers topics essential for implementing AI in healthcare responsibly: quantifying uncertainty in predictions, estimating causal (treatment) effects from observational data, domain adaptation and multi-hospital modeling for externally valid models and explainable AI.
Transferring clinical prediction models across hospitals and electronic health record systems
Curth A, Thoral P, van den Wildenberg W, Bijlstra P, de Bruin D, Elbers P, Fornasa M.
Joint European Conference on Machine Learning and Knowledge Discovery in Databases 2019 Sep 16 (pp. 605-621).
Out-of-Distribution Detection for Medical Applications: Guidelines for Practical Evaluation
Zadorozhny, K., Thoral, P., Elbers, P., & Cinà, G. (2022).
Multimodal AI in healthcare: A paradigm shift in health intelligence (pp. 137-153).
Know your limits: Uncertainty estimation with relu classifiers fails at reliable ood detection
Ulmer, D, and G Cinà.
Uncertainty in Artificial Intelligence. PMLR, 2021.
Trust Issues: Uncertainty Estimation Does Not Enable Reliable OOD Detection On Medical Tabular Data
Ulmer, D., Meijerink, L., & Cinà, G.
Machine Learning for Health (pp. 341-354) 2020. PMLR.
Uncertainty Estimation for Classification and Risk Prediction on Medical Tabular Data
Meijerink, L., Cinà, G., & Tonutti, M.
arXiv preprint arXiv:2004.05824 2020.
Bayesian Modelling in Practice: Using Uncertainty to Improve Trustworthiness in Medical Applications
Ruhe, D., Cina, G., Tonutti, M., de Bruin, D., & Elbers, P.
arXiv preprint arXiv:1906.08619, 2019.
The effect of prone positioning on mechanically ventilated COVID-19 patients
Izdebski A, Thoral PJ, Lalisang RC, McHugh DM, Entjes R, van der Meer NJ, Dongelmans DA, Boelens AD, Rigter S, Hendriks SH, de Jong R.
arXiv preprint arXiv:2109.06707. 2021 Sep 14.
Kansen Voor West III project on Causal Inference
Pacmed and the department of Medical Informatics of the AmsterdamUMC and University of Amsterdam have received a grant under the Kansen Voor West III program, funded by the European Fund for Regional Development (EFRD) of the European Union, to do research on causal inference.
Intensive care physicians could gain much more value from AI decision support when not only the risk of an undesirable outcome is predicted, but when it helps to determine the best intervention trajectory (by predicting the effects of, for example, letting a patient stay an extra day on the ICU). This requires treatment effect estimation. Techniques to estimate treatment effects come from the domain of causal inference, also referred to as causal AI.
Pacmed's collaboration with AmsterdamUMC, the Santeon hospitals and healthcare insurers such as CZ and Zilveren Kruis, will enable the validation and application of this new type of technology in practice. The project started in 2022 and will run for at least 3 years.
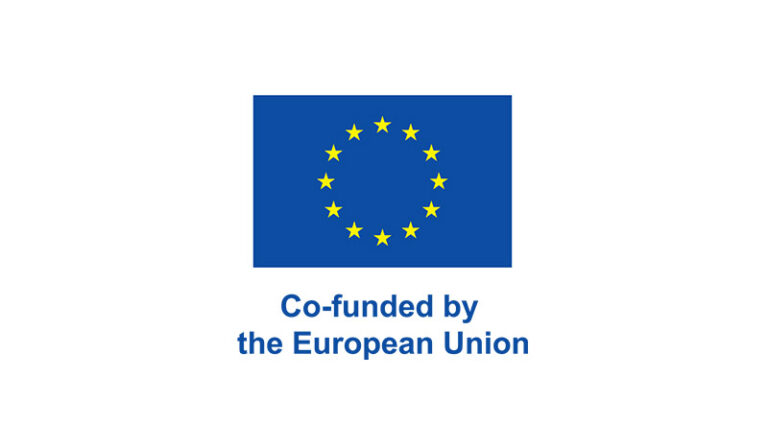
Other past work
Using machine learning to predict blood culture outcomes in the emergency department
Boerman AW, Schinkel M, Meijerink L, van den Ende ES, Pladet LC, Scholtemeijer MG, Zeeuw J, van der Zaag AY, Minderhoud TC, Elbers PW, Wiersinga WJ.
BMJ open. 2022 Jan 1;12(1):e053332.
Ultrasensitive prostate‐specific antigen level as a predictor of biochemical progression after robot‐assisted radical prostatectomy
Grivas N, de Bruin D, Barwari K, van Muilekom E, Tillier C, van Leeuwen PJ, Wit E, Kroese W, van der Poel H.
Journal of clinical laboratory analysis. 2019 Feb; 33(2):e22693.
Association of metastatic pattern in breast cancer with tumor and patient-specific factors: a nationwide autopsy study using artificial intelligence
Kazemzadeh F, Snoek JAA, Voorham QJ, van Oijen MGH, Hugen N, Nagtegaal ID.
Breast Cancer. 2024 Mar;31(2):263-271.
Generalizable calibrated machine learning models for real-time atrial fibrillation risk prediction in ICU patients
Verhaeghe J, De Corte T, Sauer CM, Hendriks T, Thijssens OWM, Ongenae F, Elbers P, De Waele J, Van Hoecke S.
International Journal of Medical Informatics. 2023 Jul;175:105086